Graph Neural Networks (GNNs): Foundation, Frontiers and Applications
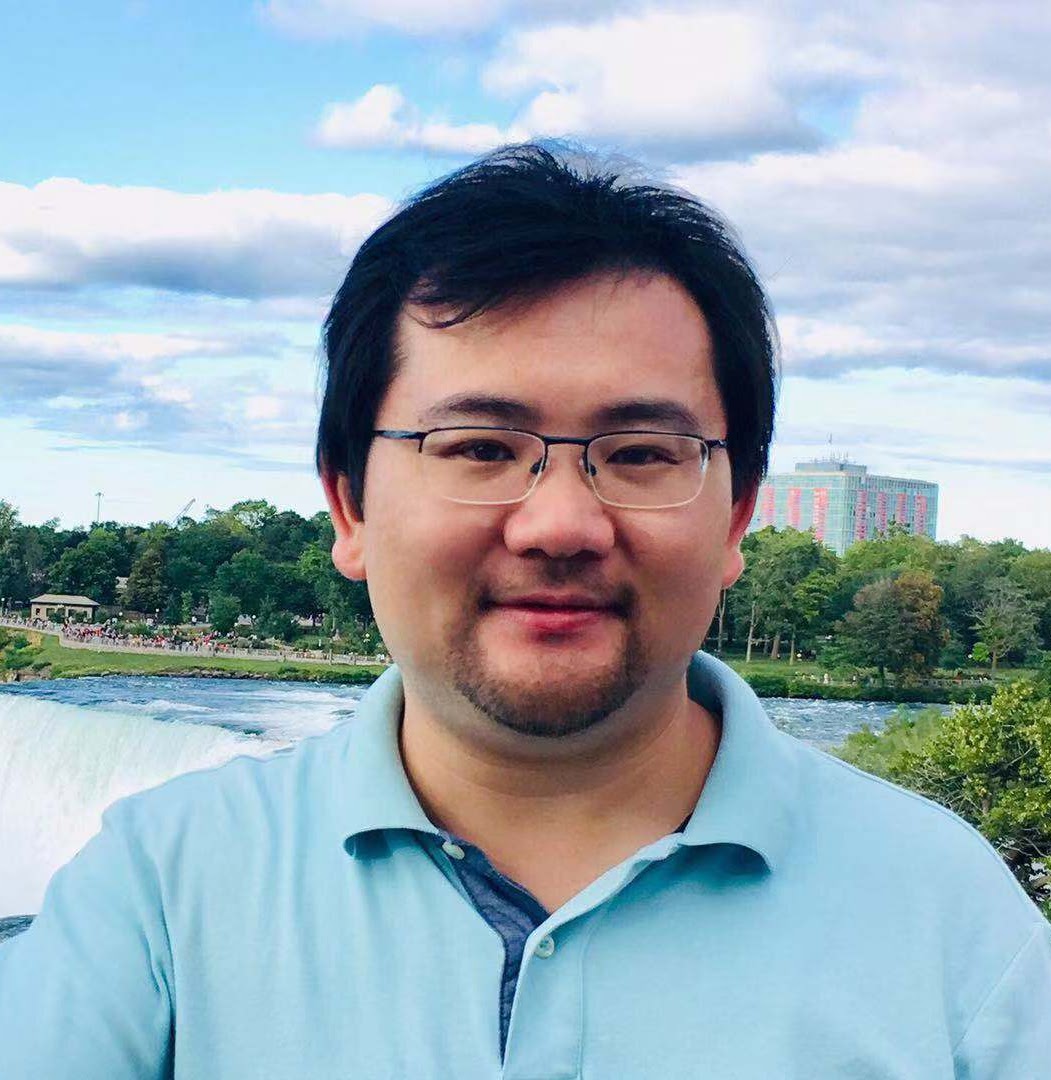
Lingfei Wu, JD.COM Silicon Valley Research Center
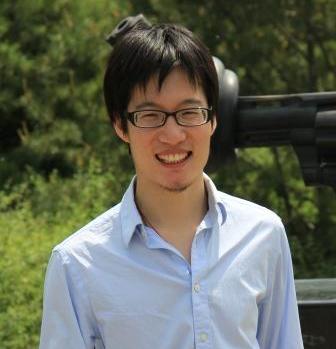
Peng Cui, Tsinghua University
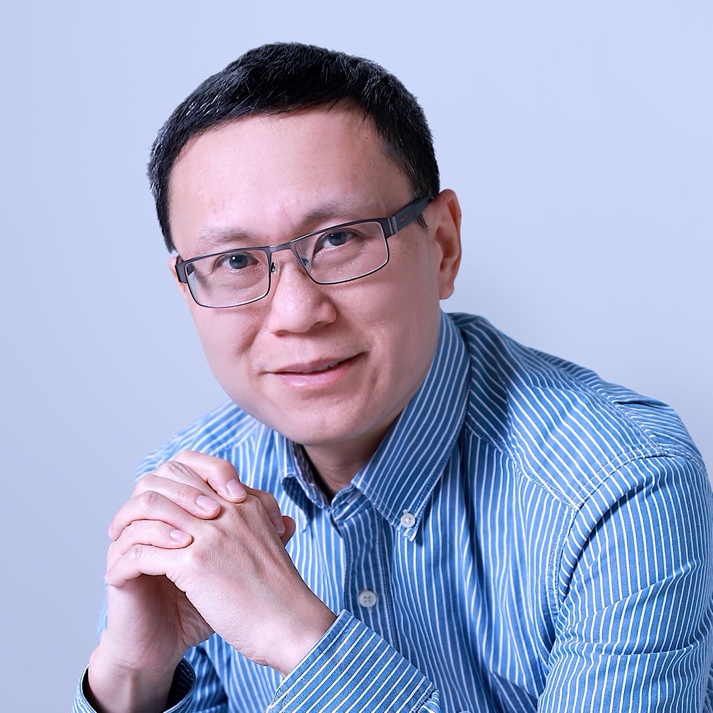
Jian Pei, Simon Fraser University
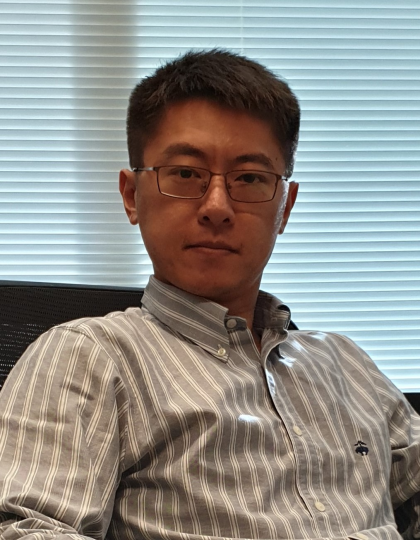
Liang Zhao, Emory University
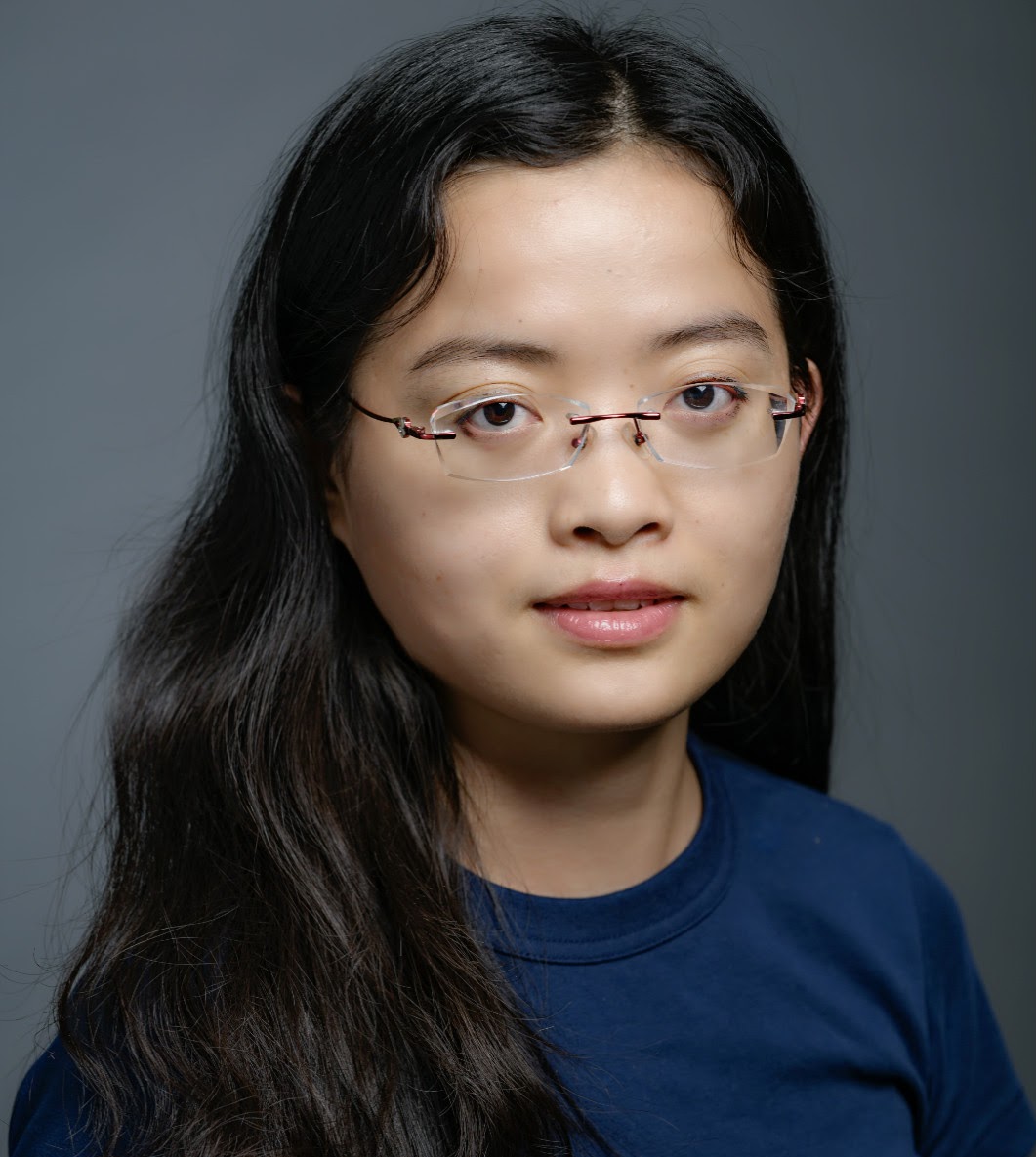
Xiaojie Guo, JD.COM Silicon Valley Research Center
Abstract
The field of graph neural networks (GNNs) has seen rapid and incredible strides over the recent years. Graph neural networks, also known as deep learning on graphs, graph representation learning, or geometric deep learning, have become one of the fastest-growing research topics in machine learning, especially deep learning. This wave of research at the intersection of graph theory and deep learning has also influenced other fields of science, including recommendation systems, computer vision, natural language processing, inductive logic programming, program synthesis, software mining, automated planning, cybersecurity, and intelligent transportation. However, as the field rapidly grows, it has been extremely challenging to gain a global perspective of the developments of GNNs. Therefore, we feel the urgency to bridge the above gap and have a comprehensive tutorial on this fast-growing yet challenging topic.
This tutorial of Graph Neural Networks (GNNs): Foundation, Frontiers and Applications will cover a broad range of topics in graph neural networks, by reviewing and introducing the fundamental concepts and algorithms of GNNs, new research frontiers of GNNs, and broad and emerging applications with GNNs. In addition, rich tutorial materials wil be included and introduced to help the audience gain a systematic understanding by using our recently published book-Graph Neural Networks (GNN): Foundation, Frontiers and Applications, one of the most comprehensive book for researchers and practitioners for reading and studying in GNNs
Outline [Slides]
Opening Remark (10 mins)- Graph Neural Networks for Node Classification
- The Express Power of Graph Neural Networks
- The Interpretability of Graph Neural Networks
- Graph Generation and Transformation
- Dynamic Graph Neural Networks
- Graph Matching
- Graph Structure Learning
- GNNs in Predicting Protein Function and Interactions
- GNNs in Graph Neural Networks in Program Analysis
- GNNs in Natural Language Processing
Organizers
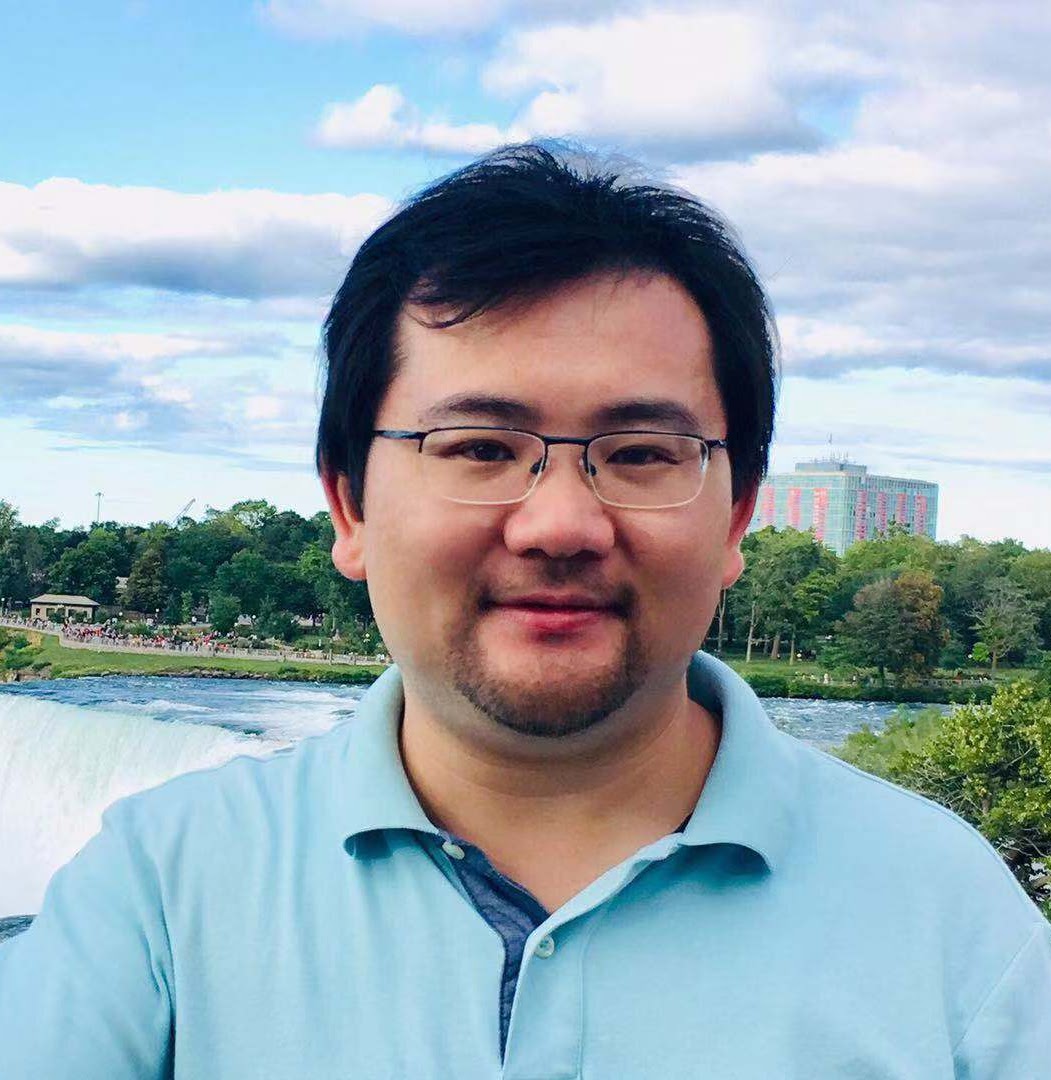
Lingfei Wu, JD.COM Silicon Valley Research Center
Lingfei Wu earned his Ph.D. degree in computer science from the College of William and Mary in 2016. He currently serves as a Principal Scientist at JD.COM Silicon Valley Research Center. He has published more than 80 top-ranked conference and journal papers and is a co-inventor of more than 40 filed US patents. Because of the high commercial value of his patents, he has received several invention achievement awards and has been appointed as IBM Master Inventors, class of 2020. He was the recipients of the Best Paper Award and Best Student Paper Award of several conferences such as IEEE ICC'19, AAAI workshop on DLGMA'20 and KDD workshop on DLG'19. His research has been featured in numerous media outlets, including NatureNews, YahooNews, Venturebeat, and TechTalks. He has co-organized 10+ conferences (KDD, AAAI, IEEE BigData) and is the founding co-chair for Workshops of Deep Learning on Graphs (with AAAI'21, AAAI'20, KDD'20, KDD'19, and IEEE BigData'19). He has currently served as Associate Editor for IEEE Transactions on Neural Networks and Learning Systems, ACM Transactions on Knowledge Discovery from Data and International Journal of Intelligent Systems, and regularly served as a SPC/PC member of the following major AI/ML/NLP conferences including KDD, IJCAI, AAAI, NIPS, ICML, ICLR, and ACL. 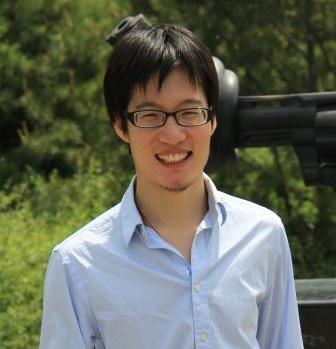
Peng Cui, Tsinghua University
Peng Cui is an Associate Professor with tenure at Department of Computer Science in Tsinghua University. He obtained his PhD degree from Tsinghua University in 2010. His research interests include data mining, machine learning and multimedia analysis, with expertise on network
https://sites.google.com/view/ representation learning, causal inference and stable learning, social dynamics modeling, and user behavior modeling, etc. He is keen to promote the convergence and integration of causal inference and machine learning, addressing the fundamental issues of today's AI technology, including explainability, stability and fairness issues. He is recognized as a Distinguished Scientist of ACM, Distinguished Member of CCF and Senior Member of IEEE. He has published more than 100 papers in prestigious conferences and journals in machine learning and data mining. He is one of the most cited authors in network embedding. A number of his proposed algorithms on network embedding generate substantial impact in academia and industry. His recent research won the IEEE Multimedia Best Department Paper Award, IEEE ICDM 2015 Best Student Paper Award, IEEE ICME 2014 Best Paper Award, ACM MM12 Grand Challenge Multimodal Award, MMM13 Best Paper Award, and were selected into the Best of KDD special issues in 2014 and 2016, respectively. He was PC co-chair of CIKM2019 and MMM2020, SPC or area chair of ICML, KDD, WWW, IJCAI, AAAI, etc., and Associate Editors of IEEE TKDE (2017-), IEEE TBD (2019-), ACM TIST(2018-), and ACM TOMM (2016-) etc. He received ACM China Rising Star Award in 2015, and CCF-IEEE CS Young Scientist Award in 2018.
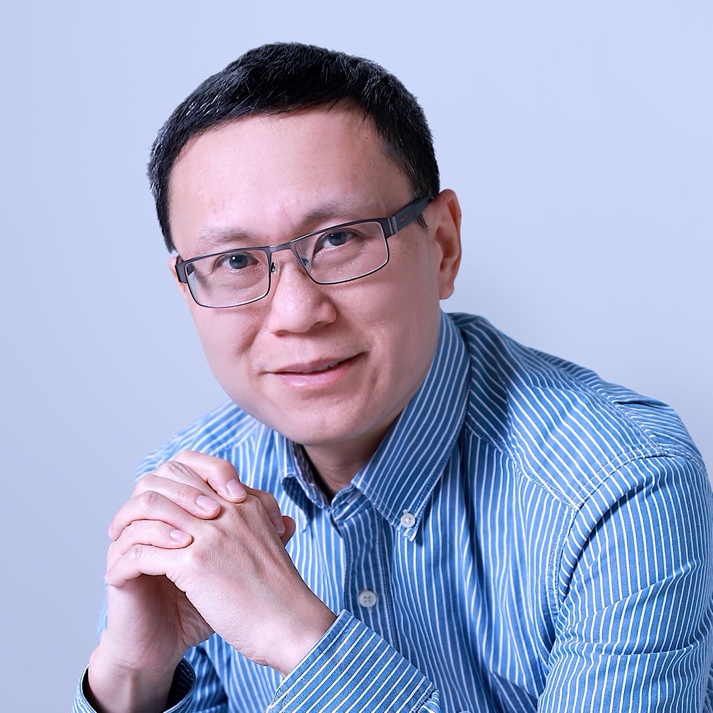
Jian Pei, Simon Fraser University
Jian Pei is a Professor in the School of Computing Science at Simon Fraser University. He is a well-known leading researcher in the general areas of data science, big data, data mining, and database systems. His expertise is on developing effective and efficient data analysis techniques for novel data intensive applications, and transferring his research results to products and business practice. He is recognized as a Fellow of the Royal Society of Canada (Canada's national academy), the Canadian Academy of Engineering, the Association of Computing Machinery (ACM) and the Institute of Electrical and Electronics Engineers (IEEE). He is one of the most cited authors in data mining, database systems, and information retrieval. Since 2000, he has published one textbook, two monographs and over 300 research papers in refereed journals and conferences, which have been cited extensively by others. His research has generated remarkable impact substantially beyond academia. For example, his algorithms have been adopted by industry in production and popular open-source software suites. Jian Pei also demonstrated outstanding professional leadership in many academic organizations and activities. He was the editor-in-chief of the IEEE Transactions of Knowledge and Data Engineering (TKDE) in 2013-16, the chair of the Special Interest Group on Knowledge Discovery in Data (SIGKDD) of the Association for Computing Machinery (ACM) in 2017-2021, and a general co-chair or program committee co-chair of many premier conferences. He maintains a wide spectrum of industry relations with both global and local industry partners. He is an active consultant and coach for industry on enterprise data strategies, healthcare informatics, network security intelligence, computational finance, and smart retail. He received many prestigious awards, including the 2017 ACM SIGKDD Innovation
Award, the 2015 ACM SIGKDD Service Award, the 2014 IEEE ICDM Research Contributions Award, the British Columbia Innovation Council 2005 Young Innovator Award, an NSERC 2008 Discovery Accelerator Supplements Award (100 awards cross the whole country), an IBM Faculty Award (2006), a KDD Best Application Paper Award (2008), an ICDE Influential Paper Award (2018), a PAKDD Best Paper Award (2014), a PAKDD Most Influential Paper Award (2009), and an IEEE Outstanding Paper Award (2007).
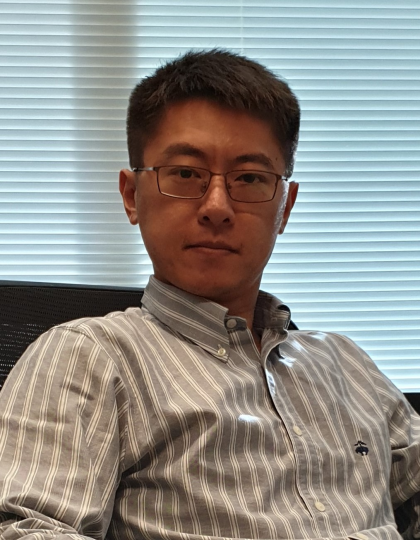
Liang Zhao, Emory University
Liang Zhao is an assistant professor at the Department of Compute Science at Emory University. Before that, he was an assistant professor in the Department of Information Science and Technology and the Department of Computer Science at George Mason University. He obtained his PhD degree in 2016 from Computer Science Department at Virginia Tech in the United States. His research interests include data mining, artificial intelligence, and machine learning, with special interests in spatiotemporal and network data mining, deep learning on graphs, nonconvex optimization, model parallelism, event prediction, and interpretable machine learning. He received AWS Machine Learning Research Award in 2020 from Amazon Company for his research on distributed graph neural networks. He won NSF Career Award in 2020 awarded by National Science Foundation for his research on deep learning for spatial networks, and Jeffress Trust Award in 2019 for his research on deep generative models for biomolecules, awarded by Jeffress Memorial Trust Foundation and Bank of America. He won the Best Paper Award in the 19th IEEE International Conference on Data Mining (ICDM 2019) for the paper of his lab on deep graph transformation. He has also won Best Paper Award Shortlist in the 27th Web Conference (WWW 2021) for deep generative models. He won Best Paper Candidate in the 21st IEEE International Conference on Data Mining (ICDM 2021) for the paper on heterogeneous graph generation by deep generative models. He was selected as “Top 20 Rising Star in Data Mining” by Microsoft Search in 2016 for his research on spatiotemporal data mining. He has also won Outstanding Doctoral Student in the Department of Computer Science at Virginia Tech in 2017. He is awarded as CIFellow Mentor 2021 by the Computing Community Consortium for his research on deep learning for spatial data. He has published numerous research papers in top-tier conferences and journals such as KDD, TKDE, ICDM, ICLR, Proceedings of the IEEE, ACM Computing Surveys, TKDD, IJCAI, AAAI, and WWW. He has been serving as organizers such as publication chair, poster chair, and session chair for many top-tier conferences such as SIGSPATIAL, KDD, ICDM, and CIKM.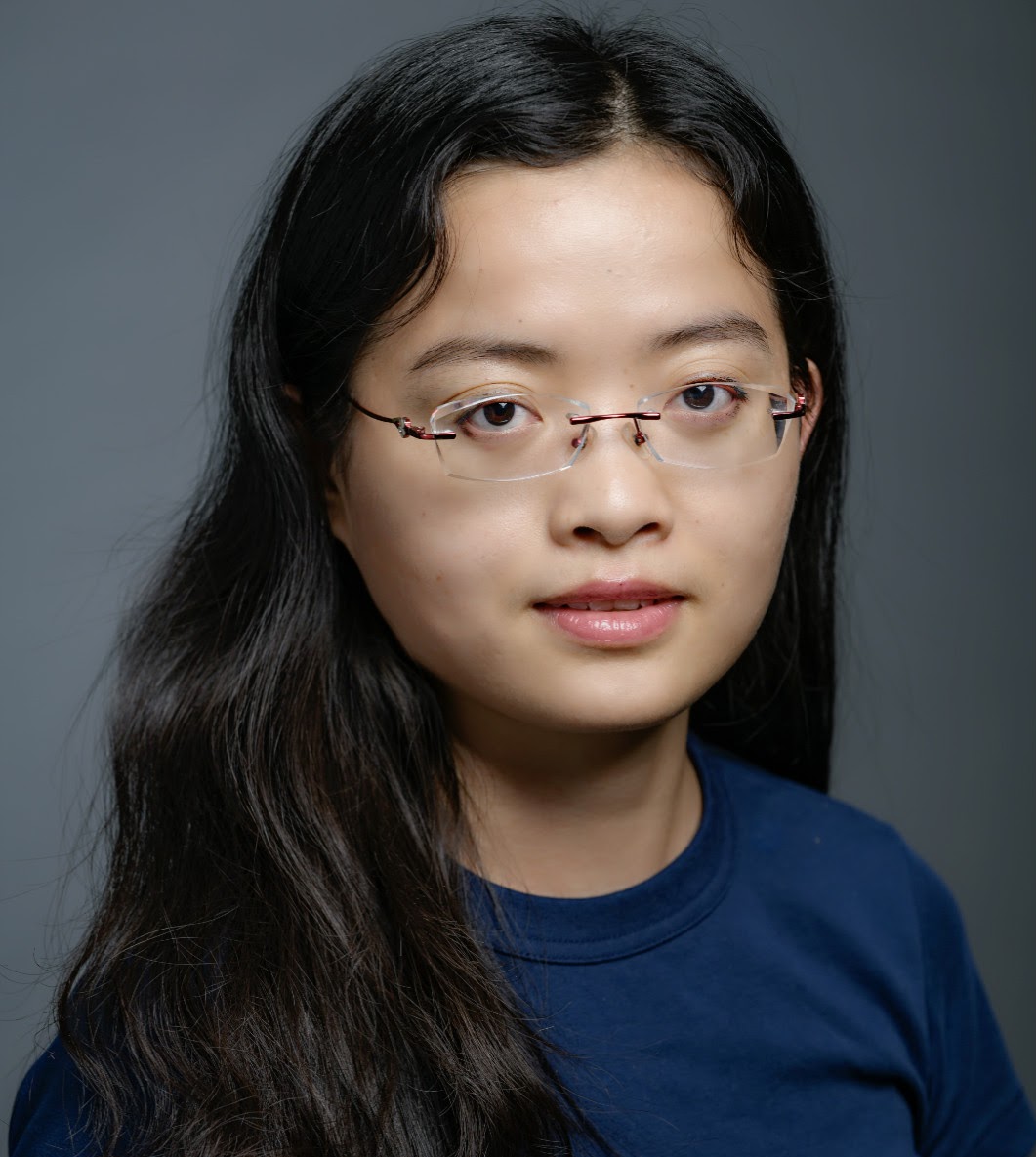